Spectral features of non-nutritive suck dynamics in extremely preterm infants
Highlight box
Key findings
• ML of Welch and Yule-Walker PSD measures revealed 3 membership classes of NNS growth patterns in EPIs born between 240/7 and 286/7 weeks’ gestation. The dependent measures peak_Hz, PSD amplitude, and AUC are highly dependent on PMA, but show little relation to respiratory status (RDS, BPD) or somatosensory intervention. These data suggest that neural regulation of the NNS compression pressure dynamics during NNS, as characterized in the frequency domain, is significantly different for each identified cluster (classes A, B, C) during this developmental period in the NICU setting.
What is known and what is new?
• Several time domain measures of NNS performance reported in the literature have been used successfully at cribside to discriminate between respiratory status (RDS, BPD) and type of somatosensory intervention delivered to EPIs in the NICU.
• The present report is the first attempt in pediatric medicine to apply ML approaches to identify the development of NNS spectral features, including changes in power spectrum density profile of suck compression bursts among cohorts of EPIs. ML algorithms revealed highly significant developmental trends in NNS burst spectra among EPIs as a function of PMA. However, the selected ML approaches did not reveal significantly different spectral profiles in these EPIs as a function of respiratory status or somatosensory intervention.
What is the implication, and what should change now?
• Efforts to refine ML algorithms to increase our knowledge of the evolution of sCPG in preterm infants, including NNS rhythmogenesis, will help us better understand the observed phenotypes of NNS burst production in both the frequency and time domains. NNS features which are relatively invariant vs. other features which are modifiable by sensory experience are likely to inform even more effective, individualized treatment strategies in this fragile population.
Introduction
The biological complexities of oral feeding make it one of the most advanced neurological milestones of the newborn and a predictor of both short- and long-term developmental outcomes in the at-risk premature neonatal population (1-5). Successful oral feeding requires the integration and coordination of oropharyngeal, respiratory and gastrointestinal systems, as well as maturation of sensory systems (visual, auditory, somatosensory, gustatory, and olfactory) and hypothalamic pathways which encode satiety and hunger (6-10). Given the adverse ex utero environment, the achievement of oral feeding competency is a universal challenge across the extremely preterm infant (EPI) population [<28 weeks gestationally-aged (GA)]. While EPIs represent only a small fraction of those born premature (11), they are most at risk for significant morbidities [e.g., necrotizing enterocolitis, intraventricular hemorrhage, and bronchopulmonary dysplasia (BPD)] that exacerbate the challenges of learning to orally feed. Due to their compromised respiratory status, EPIs who develop BPD are at greater risk for oral feeding impairments compared to GA matched infants who do not develop the disease. These infants have more difficulty achieving a coordinated suckle feeding pattern and demonstrate abnormally long periods of deglutition apnea and irregular breathing patterns during feeding (12-17). Indeed, more than 40% of children in feeding clinics were born preterm (18). In addition, premature infants who correct to term post-conceptional age (PCA) and who cannot successfully orally feed have been shown to be at increased risk for developmental disabilities (19,20) and may require surgical insertion of a gastrostomy tube to provide adequate enteral nutrition (21). Under these situations, a pacifier is often used to promote non-nutritive suck (NNS) and improve nutrition (22).
The characteristic NNS pattern is manifest as alternating epochs of burst and pause periods. A typical NNS burst consists of 6 to 12 suck cycles which are frequency-modulated (FM) with a median cycle rate at approximately 2 Hz followed by pause periods to accommodate respiration (23-25). This ororhythmic motor behavior is regulated by a heterarchically organized network consisting of a suck central pattern generator (sCPG), which includes bilateral internuncial circuits within the pontine and medullary reticular formation, descending inputs from cerebral sensorimotor areas, and modulated by a stream of sensory inputs (i.e., tactile, olfactory, gustatory) (4,9,26-29). The minimal circuitry for ororhythmic activity resides between the trigeminal motor nucleus and the facial nucleus in the brainstem (27). Thus, NNS and NS represent complex sensorimotor behaviors that can provide valuable insights into the integrity of the central nervous system (19,30).
Over the past two decades, several studies have documented time domain features of NNS structure in neurotypical term and preterm infants, and in extremely preterm newborns with respiratory complications. Measurements included identification of NNS burst-pause structure, suck compression force (pressure), suck cycle counts and rate, and more recently quantified using a statistical measure of burst structure invariance known as NNS spatiotemporal index (31-33). To date, little is known about the evolution of NNS using frequency domain analyses, including Fourier and power spectrum density measures from longitudinal sampling of NNS burst waveforms during developmental stages occurring weeks before [i.e., 30–32 weeks post-menstrual age (PMA)] and into the emergence of oral feeding skills (32–34+ weeks PMA).
The ability to suck and swallow is typically present by 28 weeks gestation, however infants are not fully coordinated until 32 to 34 weeks GA (34-36). Preterm infants born at less than 32 weeks gestation are usually not able to feed effectively or safely from the breast or a bottle. Instead, they are usually fed by a small tube that is routed through the nose and into the stomach (gavage feeding). Non-nutritive sucking on a pacifier during gavage feeding is used to promote the development of ororhythmic sucking behavior which in turn serves to improve digestion and exert a calming effect on infants. A recent Cochrane Review meta-analysis, based on 12 research trials and 746 preterm infants, indicates that NNS significantly reduces the time infants need to transition from gavage to full oral feeding, reduces the time from start of oral feeding to full oral feeding, and reduces the length of hospital stay (22).
The primary aim of this study is to model the development of NNS pressure dynamics in the frequency domain using Fourier transforms and machine learning (ML) techniques beginning at 30 weeks PMA to characterize the evolution of NNS behavior high-risk EPIs. The analyses and experimental data presented here are part of a larger ongoing study to assess the effects pulsed oral tactile stimulation on NNS development and transcriptomics of putative genes related to feeding development (ClinicalTrials.gov NCT02696343). We hypothesized that the principal frequency component and associated amplitude extracted from two select the FFT transforms (Welch method and Yule-Walker method) will show a significant dependence on PMA, and potentially modulated by the type of somatosensory intervention. Moreover, we anticipate these non-invasive and computational strategies on the spectral features of NNS production will add information to the neonatal bedside assessment of oromotor status and inform medical care decisions and improve care and outcomes regarding feeding readiness in the preterm infant. We present this article in accordance with the MDAR reporting checklist (available at https://pm.amegroups.com/article/view/10.21037/pm-21-91/rc).
Methods
Participants
Participants included 117 EPIs born between 240/7 and 286/7 weeks’ gestation, as determined by obstetric ultrasound at <15 weeks or last menstrual period. EPIs were actively enrolled once they have a corrected PMA of ≥29 weeks to limit the number of infants who develop serious sequelae of prematurity and would not be eligible for this study based upon the criteria listed below. EPI’s were recruited from four neonatal intensive care units (NICUs) including: (I) CHI Health St. Elizabeth (Lincoln, NE, USA); (II) Tufts Medical Center NICU (Boston, MA, USA); (III) Santa Clara Valley Medical Center (San Jose, CA, USA); and Children’s Hospital of Orange County (Los Angeles, CA, USA) by a study site principal/co-investigator or the neonatal study coordinator. The study was conducted in accordance with the Declaration of Helsinki (as revised in 2013). Written informed consent was obtained prior to participants’ entry into the study, following consultation with the attending physician and nurse(s) (University of Nebraska-Lincoln IRB 20150815446FB). This study is registered on ClinicalTrials.gov (NCT02696343), available at https://clinicaltrials.gov/ct2/show/NCT02696343.
Exclusion criteria: EPIs were not recruited for this study if they had any of the following: (I) chromosomal and congenital anomalies including craniofacial malformation, nervous system anomalies, cyanotic congenital heart disease, gastroschisis, omphalocele, diaphragmatic hernia and/or other major gastrointestinal anomalies; (II) congenital infection; (III) no documented GA; (IV) severe intrauterine growth retardation (IUGR) (<3%); (V) abnormal neurological status including head circumference <10th or >90th percentile, intracranial hemorrhage grades III and IV, seizures, meningitis, neurological examination showing abnormal tone or movements of all extremities for PMA; (VI) history of necrotizing enterocolitis (stage II and III); (VII) culture-positive sepsis at the time of study enrollment; and (VIII) born <24 weeks.
Intervention phase
The 117 EPIs {58 females, 59 males; GA =189.01 d [standard deviation (SD) =8.58]; PMA =212.72 d (SD =6.0 d)} were stratified among two GA groups (240/7–266/7 weeks, and 270/7–286/7 weeks). Each infant was randomized to receive either the PULSED NTrainer (n=62) or Sham (n=55) intervention. Infants assigned to the PULSED NTrainer group received a progressive dose of the pulsatile orocutaneous stimulation (37). Beginning at 30 weeks’ PMA, these infants received 2 weeks of low-dose PULSED NTrainer stimulation (2×3-minute blocks) with a 1-minute stimulus ‘off-period’ between the stimulation blocks. This form of stimulation was given simultaneous with tube feedings 3 times/day. The stimulus dose was subsequently increased over the next 2 weeks (3×3-minute blocks of PULSED NTrainer stimulation) with a 1-minute stimulus ‘off-period’ between the stimulation blocks, also given simultaneously with tube feedings 3 times/day. EPIs randomized to the Sham condition were given a regular, non-pressurized SoothieTM silicone pacifier during tube feedings over the same time period and were handled in the same manner as those infants in the experimental group of the study, with the exception of the PULSED inputs from the pacifier. EPIs were advanced on a standardized cue-based feeding schedule utilized by each site NICU, known as Infant Driven Feeding® (38,39) to promote full nipple feeds. To ensure blinding, only study site principals/co-investigators and the neonatal study coordinators were informed of infants’ treatment group assignments. Physicians, nurses, and other NICU care staff were oblivious to the treatment assignment for these study infants.
Orocutaneous stimulation regimen
The NTrainer PULSED orocutaneous stimulus consisted of a series of 6-cycle bursts which were delivered by a servo-controlled pneumatic amplifier (NTrainer SystemTM) to create rapid pressure changes within the lumen of a silicone pacifier (e.g., WeeSoothie, or regular Soothie) used in our NICUs (Figure 1). These pneumatic bursts were FM from 2.8 to 1.6 Hz across the 6-cycle structure with a 2-second pause period between bursts (25,37). A total of 34 bursts were presented in each 3-minute block. A 1-minute rest period (no stimulation) occurs between stimulation blocks. Criteria for initiation of orocutaneous therapy include the following: (I) stable vital signs and not on continuous vasopressor medications; (II) tolerating enteral feeds in previous 48 hours; and (III) not intubated and mechanically ventilated. If the infant is on nasal intermittent positive pressure ventilation, continuous positive airway pressure or nasal cannula >2 L/min, then the fraction of inspired oxygen (FiO2) must be <40%.
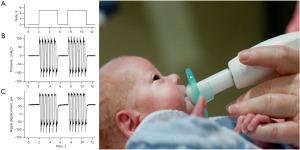
NNS assessment and automated NNS digital signal processing and feature extraction
In addition to the oral stimulation interventions (PULSED NTrainer vs. Sham pacifier), study infants were assessed 3 times/week (e.g., Mon/Wed/Fri) for NNS performance. Nipple compression dynamics during NNS was digitized during a 3-minute session immediately preceding a tube feeding not associated with an intervention trial. A cross-platform graphical user interface and terminal Python application known as NeoNNS created in our laboratory was used for batch file time series and frequency-domain analyses of NNS compression pressure waveforms on 2,133 NNS data files using analysis parameters derived from our previous research on suck dynamics (31). These parameters are summarized below.
Calibration and filter
NeoNNS was used to automatically convert NNS assessment data files digitized on the NTrainer System from voltage to cmH2O-based on a 2-point calibration algorithm. The suck pressure signal was low-pass filtered (4-pole, digital Butterworth LP® 50 Hz) to remove transients and high-frequency noise, and subsequently down-sampled to 100 samples/second to improve memory resource management and computational throughput while preserving the fidelity of NNS waveform features for discrimination consistency.
Baseline correction pipeline
NNS pressure signals are susceptible to thermal drift because of oral heat transfer from the neonate’s mouth to the silicone pacifier. If left uncorrected, this could impact the accuracy of NNS burst discrimination. Baseline variation is an important issue in many signal processing applications and can be addressed using baseline estimation or correction methods. NeoNNS benefits from an asymmetric least-squares smoothing (ALSS) correction algorithm (40) to automatically correct the nipple pressure signal baseline. The ALSS algorithm effectively pulls all the lower points of every nipple pressure waveform back to the zero baseline while maintaining the structure of the suck compression waveform shape.
Suck compression peak identification methods
An automatic peak picker was designed to index and sort true-NNS pressure peaks from non-NNS events according to these rules: pressure peaks must exceed a user-defined pressure threshold (e.g., 1.6 cmH2O) and meet a specified half-height pulse width criterion (31). Discriminated NNS cycles are labeled at their peaks with a green circle, and the non-NNS cycles are labeled with a red circle (Figure 2). The most active period of the NNS output (e.g., 2 minutes) for any given data file is selected, and suck bursts are automatically extracted and indexed according to their time order. An NNS burst is defined as 2 or more suck cycles satisfying user-defined cycle periods (e.g., <1,200 ms).
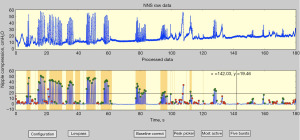
Frequency domain analytics
NNS waveforms were converted to the frequency domain using NeoNNS (31), including the fast Fourier transformation (FFT) (41), Welch’s method (42), and Yule-Walker method (43). In Welch’s method, the power spectral density (PSD) estimate is computed by dividing the 2 minutes of the NNS signal into 90% of overlapped segments and applying a 50% length flattop window to prevent the leakage effect. The Yule-Walker method estimates the PSD by fitting the autoregressive model to the windowed (nominally at 50% of overall length) time-series data with the estimation order of 8. A high pass filter (fc=0.4 Hz) was applied before spectrum calculation to remove the direct current (DC) offset.
Data analysis
A-priori power calculation indicated that a sample size of 110 was required for 80% power, with an assumed correlation of .60 among repeated measures and a small effect over time (Cohen’s f=0.10). This effect was considerably smaller than those observed in our previous empirical data (f=0.22–0.24), suggesting adequate power for the final sample of 117 EPIs in this study.
Data analysis proceeded in two stages. In the first stage, longitudinal cluster analysis was conducted to identify groups (classes) of babies, each showing a unique pattern of change in WELCHpeak_Hz over the NICU therapy phases. The unsupervised ML approach was applied for clustering as follows. First, the optimal number of classes were determined by using non-parametric criteria (44-46). Then, k-means algorithm was applied to partition individual trajectories (babies) into those identified classes (47). Given the number of classes, this algorithm finds class membership that minimize the variance within each class and maximize the variance between the classes (48).
In the second stage, linear mixed modeling (LMM) analysis was performed for the Welch and Yule-Walker dependent variables to examine the effects of GA, PMA, sex (male, female), patient type [respiratory distress syndrome (RDS), BPD], treatment (NTrainer, Sham), intervention phase [1, 2, 3], class, as well as phase-by-class interaction. The models accounted for nesting of repeated measurements within babies (i.e., intraclass correlation), and a proper error covariance structure was determined based on model fit (i.e., adjusted Akaike information criterion, Bayesian information criterion). All statistical analyses were conducted using R (49) and SAS 9.4 (50).
Results
Examples of the Welch and Yule-Walker PSD transforms are shown graphically as a three-dimensional surface (51) (rendered in 80×80 mesh) in Figures 3,4, respectively, for the same EPI from 214 to 253 days PMA. The progressive evolution of the NNS is clearly evident with peak frequencies at approximately 1.5 Hz early on and progressing to approximately 2.1 Hz by 250 days PMA. Growth in the amplitude of the spectral peaks as a function of PMA is also apparent in both PSD surface renderings. One notable difference between the Welch and Yule-Walker PSD surface renderings is the sharpness of the frequency peaks, favoring the Welch method. NNS spectral peaks using the Yule-Walker PSD method are smoother, resembling a low-pass filtered version of those produced using the Welch method with slightly different peak frequency values.
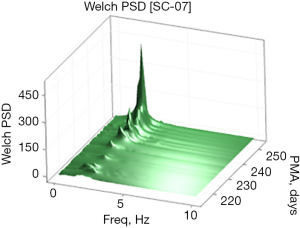
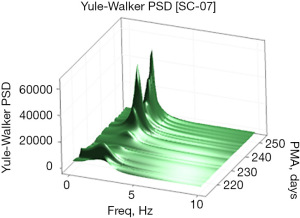
ML-based longitudinal clustering, with treatment stage as a time variable (3 time points: 30 PMA, 32 PMA, and >34 PMA), for the dependent variable WELCHpeak_Hz revealed 3 classes of babies derived from the pool of 117 EPIs who participated in this trial (Figure 5).
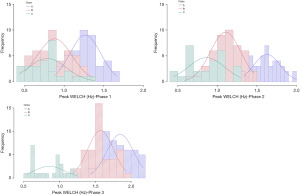
Neonate membership included class A (n=44, 25 females), class B (n=46, 20 females), and class C (n=27, 13 females), each representing a unique pattern of growth in WELCHpeak_Hz (Figure 6). Additional clinical details concerning class comparisons are given in Table 1.
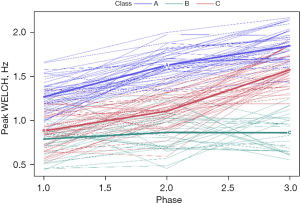
Table 1
Variables | All | A (n=44) | B (n=46) | C (n=27) | Difference | |||||||||||||
---|---|---|---|---|---|---|---|---|---|---|---|---|---|---|---|---|---|---|
N | M (%) | SD | N | M (%) | SD | N | M (%) | SD | N | M (%) | SD | P | η2 (V) | |||||
GA | 117 | 189.01 | 8.58 | 44 | 187.93 | 9.32 | 46 | 189.67 | 8.44 | 27 | 189.63 | 7.64 | 0.5777 | 0.01 | ||||
PMA (baseline) | 117 | 212.72 | 6.00 | 44 | 215.89 | 7.15 | 46 | 211.33 | 5.06 | 27 | 209.93 | 1.77 | 0.0001* | 0.18 | ||||
Sex | 0.4428 | 0.12 | ||||||||||||||||
Male | 59 | 50.4 | 19 | 16.2 | 26 | 22.2 | 14 | 12.0 | ||||||||||
Female | 58 | 49.6 | 25 | 21.4 | 20 | 17.1 | 13 | 11.1 | ||||||||||
Patient type | 0.7815 | 0.06 | ||||||||||||||||
RDS | 88 | 75.2 | 34 | 29.1 | 33 | 28.2 | 21 | 18.0 | ||||||||||
BPD | 29 | 24.8 | 10 | 8.6 | 13 | 11.1 | 6 | 5.1 | ||||||||||
Treatment | 0.3214 | 1.39 | ||||||||||||||||
NTrainer | 62 | 53.0 | 24 | 20.5 | 27 | 23.1 | 11 | 9.4 | ||||||||||
Sham | 55 | 47.0 | 20 | 17.1 | 19 | 16.2 | 16 | 13.7 |
EPI, extremely preterm infant; M, mean; SD, standard deviation; GA, gestationally-aged; PMA, post-menstrual age; RDS, respiratory distress syndrome; BPD, bronchopulmonary dysplasia.
The follow-up analysis using LMM showed that growth in WELCHpeak_Hz was significantly related to PMA (P<0.0001) and neonate classes (phase-by-class interaction P<0.0001). Patient type, sensory treatment type, and sex were not significant factors. Each neonate class showed a unique pattern of change (i.e., growth trajectory) over the NICU therapy phases, confirming the findings from the longitudinal clustering. Classes A and B showed significant positive trends (=0.16, P<0.0001 and =0.18, P<0.0001, respectively) in the principal frequency (rhythm) of NNS cycle production over the intervention phases. The 95% confidence intervals (CIs) of the trajectories, as depicted by the shaded areas in Figure 7, confirm a significant difference between classes A and B neonates. Overall, babies in class A manifest an NNS WELCHpeak_Hz suck cycle rhythm that was 0.42 Hz higher than babies in class B during the intervention. At the beginning of the intervention phase, NNS WELCHpeak_Hz was approximately 1.40 Hz for class A neonates and finished at 1.71 Hz by the end of intervention, whereas class B neonates started NNS WELCHpeak_Hz at 1.00 Hz and increased to 1.36 Hz at the end of intervention. Neonates in class C shared a similar starting point in NNS WELCHpeak_Hz at 0.97 Hz but showed no significant growth (=−0.06, P=0.06) in this spectral measure over the 4-week intervention which spanned from 30 weeks PMA to 34 weeks PMA. Moreover, although classes B and C neonates showed a similar NNS WELCHpeak_Hz at the start of intervention, class B neonates manifest a significant divergence from their class C counterparts as shown by the 95% CIs with advancement through the intervention phase.
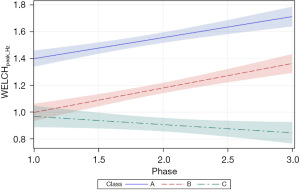
Two additional Welch dependent variables were examined, including PSD amplitude (WELCHPSD_amp), and Welch area under the curve (AUC) (WELCHAUC). For WELCHPSD_amp, significant positive slopes were found for neonates in classes A and B (=45.71, P<0.0001 and =29.86, P<0.01, respectively). The magnitude of the WELCHPSD_amp for neonates in classes A and B nearly doubled from phase 1 to phase 3 of the intervention in the NICU. Neonates within the class C cluster manifest the lowest starting WELCHPSD_amp values (60.11) with no apparent change during the intervention and confirmed by a non-significant slope in the growth function (=−10.33, P=0.36). As shown in Figure 8, the 95% CIs overlap considerably during the early phases of intervention, with only classes A and C showing a significant separation beginning at phase 1.5 of intervention.
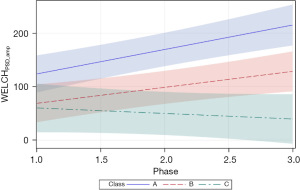
The final Welch variable examined, WELCHAUC, revealed a pattern of growth similar to WELCHPSD_amp with significant positive slopes were observed for neonates in classes A and B (=23.48, P<0.0001 and =17.89, P<0.0001, respectively). The WELCHAUC measure for neonates in classes A and B approximately doubled from phase 1 to 3 of the intervention in the NICU. Neonates of the class C cluster manifest the lowest starting WELCHAUC values (29.64) with no apparent change during the intervention and confirmed by a non-significant slope in the growth function (=−5.04, P=0.3253). As shown in Figure 9, the 95% CIs overlap considerably during the early phases of intervention, with only classes A and C showing a significant separation beginning at phase 1.5 of intervention.
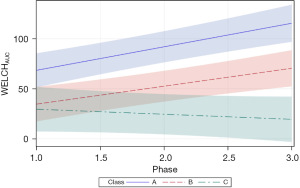
Growth in the YULE_WALKERpeak_Hz autocorrelation measure was significantly related to PMA (P<0.0001) and neonate classes (phase-by-class interaction P<0.0001). Patient type, sensory treatment type, and sex were not significant factors. Each neonate class showed a unique growth trajectory over the NICU therapy phases. Classes A and B showed significant positive growth trends in the principal frequency (rhythm) of NNS cycle production over the 4-week intervention phase and were significantly different from one another (Figure 10). Overall, the slope for the YULE_WALKERpeak_Hz growth function in class B neonates was twice that observed in class A neonates (=0.18, P<0.0001 and =0.36, P<0.0001, respectively). Class A neonates manifest a baseline YULE_WALKERpeak_Hz NNS rate of 1.56 Hz, compared to classes B and C neonates at 0.90 and 0.79 Hz, respectively. No significant growth in YULE_WALKERpeak_Hz was observed in the NNS waveforms among class C neonates (=−0.002, P=0.96) during the sensory intervention, whereas class B neonates showed positive growth in the principal NNS cycle frequency which converged towards class A neonates near the end of the intervention phase.
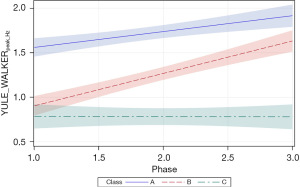
Two additional features of the Yule-Walker power spectrum were examined, including PSD amplitude (YULE_WALKERPSD_amp) and Yule-Walker AUC (YULE_WALKERAUC). These measures were significantly related to PMA (both P<0.01) and therapy phase (P<0.05 and P<0.01, respectively). Patient type, sensory treatment type, and sex were not significant factors. Unlike the corresponding Welch measures, significant positive slopes were found for neonates in all 3 cluster classes. The positive growth in YULE_WALKERPSD_amp for classes A, B, and C are given in the corresponding slopes (=6,646.20, P<0.0001, =5,050.82, P<0.001, and =3,249.24, P<0.05, respectively) (Figure 11). Likewise, the positive growth in YULE_WALKERAUC for each class is given by =4,192.86, P<0.01, =6,141.23, P<0.0001, and =4,589.22, P<0.01, respectively (Figure 12). Closer inspection of the 95% CIs shows class A YULE_WALKERAUC values non-overlapping with higher predicted y values compared to classes B and C which show considerable overlap throughout the sensory intervention phases.
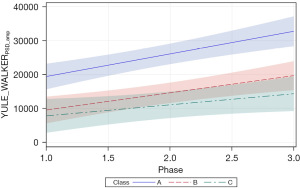
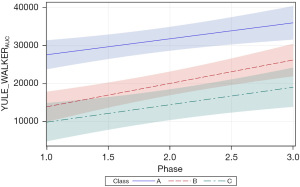
Discussion
The two spectral analysis methods we explored, Welch and Yule-Walker PSD, revealed 3 classes of EPI growth patterns in NNS development. The dependent measures derived from each spectral method (peak_Hz, PSD amplitude, and AUC) are highly dependent on PMA, but show little relation to respiratory status (RDS, BPD) or somatosensory intervention from 30 weeks PMA until discharge from the NICU. This suggests that the frequency controller of sCPG for EPIs, as discriminated by our ML cluster analysis, is remarkably stable and significantly different for each of the identified clusters (classes A, B, C) and predictable during this developmental period. These differences and trends are considered in more detail below.
Of the three dependent measures, peak_Hz showed the lowest variance with tighter 95% CIs among the three EPI class clusters during the sensory intervention phases. peak_Hz estimates tended to be somewhat higher for the Yule-Walker method compared to the Welch method. EPI class A yielded the highest peak_Hz values using either spectral method and was clearly distinct from classes B and C. Overall, EPIs with membership in class A exhibited the greatest spectral peak_Hz for NNS data sampled at the beginning of the sensory intervention, ranging from 1.40 Hz (Welch) to 1.56 Hz (Yule-Walker) and increased to 1.72 Hz (Welch) and 1.92 Hz (Yule-Walker) by phase 3 of the sensory intervention. EPIs in class B also showed significant positive trends in NNS peak_Hz but started at a lower frequency 1.0 and 0.9 Hz, for Welch and Yule-Walker methods, respectively. The class B peak_Hz function paralleled peak_Hz levels attained by EPIs in class A, with a negative offset of approximately 0.4 Hz. Interestingly, EPIs with membership in class C manifest no significant change in peak_Hz over the sensory intervention phase, with no dependence on respiratory status, or sensory type.
The metric, PSD amplitude for both methods (WELCHPSD_amp, YULE-WALKERPSD_amp), was less discriminative compared to the peak_Hz measure. The YULE-WALKERPSD_amp was more discriminative for class A vs. classes B and C, with clear separation in their respective 95% CIs. YULE-WALKERAUC resulted in positive growth as a function of sensory intervention phase for EPIs among the three classes, with significant separation between class A and classes B and C. The integration of spectral energy represented by the AUC calculation can be interpreted as growth in NNS performance from 30 weeks PMA until discharge from the NICU. The WELCHAUC showed positive growth for EPIs with membership in classes A and B, whereas a slight negative slope was observed for this measure in class C EPIs. Class separation was apparent during stage 3 of sensory intervention.
This is the first study to apply Fourier analytics to derive PSD measures using the Welch and Yule-Walker methods to increase our understanding of NNS dynamics and motor control in EPIs, carefully documented for respiratory status and somatosensory intervention initiated at 30 weeks PMA. LMM revealed a strong relationship between PMA and the three spectrally dependent measures. ML-based longitudinal clustering, with treatment stage as a time variable, revealed 3 classes of babies derived from the pool of 117 EPIs who participated in this trial. However, the underlying factors and/or developmental mechanisms contributing to these 3 resultant classes remains unknown. Clearly, EPIs with membership in classes A and B showed significant positive trends peak_Hz, PSD_amp, and PSD_AUC, with class A performing at a higher level of output during NNS production. Contrary to our initial predictions, respiratory status (BPD, RDS) and sensory intervention type (NTrainer, Sham) were not significant factors to account for the various EPI classifications (A, B, C). Thus, the fundamental rhythm and spectral ‘signature’ of the NNS may be a product of early in utero prenatal experiences, and/or determined by genetic mechanisms that are expressed in EPIs as a relatively stable motor behavior, at least in the frequency domain.
Recent evidence on the relative invariance of the fundamental rhythm of NNS production in infants is based on simple suck cycle counts per unit time. NNS is regarded as a biomarker of neonatal sensorimotor development, reflecting the integrity of the nervous system and progression telltale in oromotor development (19). For example, between 3 and 12 months of age, NNS duration, number of bursts, suck compression cycles/burst significantly decrease while suck compression pressure amplitude significantly increases over the same time period (52). In contrast to these event count metrics, the median frequency of suck cycles within frequency modulated bursts remains relatively constant (~2.1 Hz) (53). For example, 3-month-old infants produced a median of 4.5 suck bursts per minute with 9.60 cycles/burst at a mean amplitude of 14.05 cmH2O, resulting in an NNS burst duration of 4.74 s. In contrast, 12-month-old infants produced a median of 2.5 suck bursts per minute with 3.75 cycles/burst at a mean amplitude of 19.75 cmH2O, resulting in a burst duration of 1.67 s. Thus, as the infant progresses through the first year their NNS bursts become fewer in number and shorter, but with 40% greater suck compression amplitudes (52).
Impairments in NNS rhythm and patterning have been correlated to prenatal maternal exposure to specific airborne particular matter components in Puerto Rican infants soon after birth (54). Reductions in NNS cycle frequency and changes in suck compression amplitude are also associated with gestational exposure to phthalates [chemicals found in personal care products, polyvinyl chloride (PVC) plastics, and other products]. Under these circumstances, assessment of NNS dynamics using spectral analytics may have important clinical implications for early detection of toxin exposure-related deficits in sCPG function as well as implications for subsequent neurodevelopment and individualized interventions for the infant with a feeding disorder (55).
Oral feeding is a complex neurological milestone that can pose a significant challenge for many preterm infants, particularly for those born extremely premature (<28 weeks GA). Efforts to increase our knowledge of the evolution of the sCPG in preterm infants, including the biomechanics and development of NNS rhythmogenesis will help us better understand the observed phenotypes of NNS production in both the frequency and time domain. Knowledge of those features of the NNS which are relatively invariant vs. other features which are modifiable by experience will likewise inform more effective treatment strategies in this fragile population. Two major lines of ongoing research in our laboratories and NICUs are exploring predictors of feeding readiness, including gene transcriptomics of putative feeding circuits (10,37,56,57), and motor control of ororhythmic pattern formation during NNS (37). For example, hierarchical cluster and feature analyses of NNS force dynamics have shown promise as a predictor of feeding readiness in EPIs (31). The inclusion of gene transcriptomics is expected to elucidate new factors to better understand the origins of the EPI membership classes discovered in the present report using machine language analytics.
Acknowledgments
Funding: This research was funded by the Eunice Kennedy Shriver National Institutes of Child Health and Human Development (R01 HD086088).
Footnote
Provenance and Peer Review: This article was commissioned by the editorial office, Pediatric Medicine for the series “Neonatal Feeding and Developmental Issues”. The article has undergone external peer review.
Reporting Checklist: The authors have completed the MDAR reporting checklist. Available at https://pm.amegroups.com/article/view/10.21037/pm-21-91/rc
Data Sharing Statement: Available at https://pm.amegroups.com/article/view/10.21037/pm-21-91/dss
Peer Review File: Available at https://pm.amegroups.com/article/view/10.21037/pm-21-91/prf
Conflicts of Interest: Authors have completed the ICMJE uniform disclosure form (available at https://pm.amegroups.com/article/view/10.21037/pm-21-91/coif). JPC passed away recently, therefore, his COI form was not collected. The series “Neonatal Feeding and Developmental Issues” was commissioned by the editorial office without any funding or sponsorship. SMB is the primary inventor of the NTrainer System. This intellectual property is managed by the Kansas University Technology Center. Also, SMB served as the unpaid Guest Editor of the series and serves as an unpaid editorial board member of Pediatric Medicine from July 2021 to June 2024. BG reported consulting fees from Innara Health in 2021 and got support for attending meetings and/or travel from Innara Health in 2022. The authors have no other conflicts of interest to declare.
Ethical Statement: The authors are accountable for all aspects of the work in ensuring that questions related to the accuracy or integrity of any part of the work are appropriately investigated and resolved. The study was conducted in accordance with the Declaration of Helsinki (as revised in 2013). The study was approved by human subjects institutional review board of the University of Nebraska-Lincoln (No. 20150815446FB) and written informed consent was taken from all individual participants.
Open Access Statement: This is an Open Access article distributed in accordance with the Creative Commons Attribution-NonCommercial-NoDerivs 4.0 International License (CC BY-NC-ND 4.0), which permits the non-commercial replication and distribution of the article with the strict proviso that no changes or edits are made and the original work is properly cited (including links to both the formal publication through the relevant DOI and the license). See: https://creativecommons.org/licenses/by-nc-nd/4.0/.
References
- Lau C, Alagugurusamy R, Schanler RJ, et al. Characterization of the developmental stages of sucking in preterm infants during bottle feeding. Acta Paediatr 2000;89:846-52. [Crossref] [PubMed]
- Delaney AL, Arvedson JC. Development of swallowing and feeding: prenatal through first year of life. Dev Disabil Res Rev 2008;14:105-17. [Crossref] [PubMed]
- Adams-Chapman I, Bann CM, Vaucher YE, et al. Association between feeding difficulties and language delay in preterm infants using Bayley Scales of Infant Development-Third Edition. J Pediatr 2013;163:680-5.e1-3.
- Barlow SM, Rosner A, Song D. Feeding and Brain Development in Preterm Infants: Central Pattern Generation and Suck Dynamics. In: Govindaswami B. editor. Preventive Newborn Health: A Global Approach in the Age of Information. New Delhi: Jaypee Brothers Medical Publishers, 2021:255-63.
- Barlow SM, Rosner A, Song D. Feeding and Brain Development in Preterm Infants: Role of Sensory Stimulation. In: Govindaswami B. editor. Preventive Newborn Health: A Global Approach in the Age of Information. New Delhi: Jaypee Brothers Medical Publishers, 2021:264-74.
- Panksepp J. Hypothalamic regulation of energy balance and feeding behavior. Fed Proc 1974;33:1150-65. [PubMed]
- King BM. The rise, fall, and resurrection of the ventromedial hypothalamus in the regulation of feeding behavior and body weight. Physiol Behav 2006;87:221-44. [Crossref] [PubMed]
- Verhagen JV, Engelen L. The neurocognitive bases of human multimodal food perception: sensory integration. Neurosci Biobehav Rev 2006;30:613-50. [Crossref] [PubMed]
- Schaal B. Mammary odor cues and pheromones: mammalian infant-directed communication about maternal state, mammae, and milk. Vitam Horm 2010;83:83-136. [Crossref] [PubMed]
- Maron JL, Hwang JS, Pathak S, et al. Computational gene expression modeling identifies salivary biomarker analysis that predict oral feeding readiness in the newborn. J Pediatr 2015;166:282-8.e5. [Crossref] [PubMed]
- Martin JA, Osterman MJ, Sutton PD. Are preterm births on the decline in the United States? Recent data from the National Vital Statistics System. NCHS Data Brief 2010;1-8. [PubMed]
- Gewolb IH, Bosma JF, Taciak VL, et al. Abnormal developmental patterns of suck and swallow rhythms during feeding in preterm infants with bronchopulmonary dysplasia. Dev Med Child Neurol 2001;43:454-9. [Crossref] [PubMed]
- Gewolb IH, Bosma JF, Reynolds EW, et al. Integration of suck and swallow rhythms during feeding in preterm infants with and without bronchopulmonary dysplasia. Dev Med Child Neurol 2003;45:344-8. [Crossref] [PubMed]
- da Costa SP, van der Schans CP, Zweens MJ, et al. Development of sucking patterns in pre-term infants with bronchopulmonary dysplasia. Neonatology 2010;98:268-77. [Crossref] [PubMed]
- Howe TH, Sheu CF, Holzman IR. Bottle-feeding behaviors in preterm infants with and without bronchopulmonary dysplasia. Am J Occup Ther 2007;61:378-83. [Crossref] [PubMed]
- Mizuno K, Nishida Y, Taki M, et al. Infants with bronchopulmonary dysplasia suckle with weak pressures to maintain breathing during feeding. Pediatrics 2007;120:e1035-42. [Crossref] [PubMed]
- Martin-Harris B. Clinical implications of respiratory-swallowing interactions. Curr Opin Otolaryngol Head Neck Surg 2008;16:194-9. [Crossref] [PubMed]
- Lau C. Oral feeding in the preterm infant. Neoreviews 2006;7:e19-27. [Crossref]
- Mizuno K, Ueda A. Neonatal feeding performance as a predictor of neurodevelopmental outcome at 18 months. Dev Med Child Neurol 2005;47:299-304. [Crossref] [PubMed]
- Samara M, Johnson S, Lamberts K, et al. Eating problems at age 6 years in a whole population sample of extremely preterm children. Dev Med Child Neurol 2010;52:e16-22. [Crossref] [PubMed]
- Wilson L, Oliva-Hemker M. Percutaneous endoscopic gastrostomy in small medically complex infants. Endoscopy 2001;33:433-6. [Crossref] [PubMed]
- Foster JP, Psaila K, Patterson T. Non-nutritive sucking for increasing physiologic stability and nutrition in preterm infants. Cochrane Database Syst Rev 2016;10:CD001071. [Crossref] [PubMed]
- Wolff PH. The serial organization of sucking in the young infant. Pediatrics 1968;42:943-56. [Crossref] [PubMed]
- Finan DS, Barlow SM. The actifier: a device for neurophysiological studies of orofacial control in human infants. J Speech Hear Res 1996;39:833-8. [Crossref] [PubMed]
- Barlow SM, Burch M, Venkatesan L, et al. Frequency Modulation and Spatiotemporal Stability of the sCPG in Preterm Infants with RDS. Int J Pediatr 2012;2012:581538. [Crossref] [PubMed]
- Iriki A, Nozaki S, Nakamura Y. Feeding behavior in mammals: corticobulbar projection is reorganized during conversion from sucking to chewing. Brain Res Dev Brain Res 1988;44:189-96. [Crossref] [PubMed]
- Tanaka S, Kogo M, Chandler SH, et al. Localization of oral-motor rhythmogenic circuits in the isolated rat brainstem preparation. Brain Res 1999;821:190-9. [Crossref] [PubMed]
- Barlow SM, Finan DS, Lee J, et al. Synthetic orocutaneous stimulation entrains preterm infants with feeding difficulties to suck. J Perinatol 2008;28:541-8. [Crossref] [PubMed]
- Barlow SM, Lund JP, Estep M, et al. Central pattern generators for speech and orofacial activity. In: Brudzynski SM. editor. Handbook of mammalian vocalization: an integrative neuroscience approach. 1st ed. Oxford: Elsevier, 2010:351-70.
- Barlow SM, Estep M. Central pattern generation and the motor infrastructure for suck, respiration, and speech. J Commun Disord 2006;39:366-80. [Crossref] [PubMed]
- Liao C, Rosner AO, Maron JL, et al. Automatic Nonnutritive Suck Waveform Discrimination and Feature Extraction in Preterm Infants. Comput Math Methods Med 2019;2019:7496591. [Crossref] [PubMed]
- Poore M, Barlow SM, Wang J, et al. Respiratory treatment history predicts suck pattern stability in preterm infants. J Neonatal Nurs 2008;14:185-92. [Crossref] [PubMed]
- Poore M, Zimmerman E, Barlow SM, et al. Patterned orocutaneous therapy improves sucking and oral feeding in preterm infants. Acta Paediatr 2008;97:920-7. [Crossref] [PubMed]
- Hack M, Estabrook MM, Robertson SS. Development of sucking rhythm in preterm infants. Early Hum Dev 1985;11:133-40. [Crossref] [PubMed]
- Barlow SM. Central pattern generation involved in oral and respiratory control for feeding in the term infant. Curr Opin Otolaryngol Head Neck Surg 2009;17:187-93. [Crossref] [PubMed]
- Barlow SM. Oral and respiratory control for preterm feeding. Curr Opin Otolaryngol Head Neck Surg 2009;17:179-86. [Crossref] [PubMed]
- Barlow SM, Maron JL, Alterovitz G, et al. Somatosensory Modulation of Salivary Gene Expression and Oral Feeding in Preterm Infants: Randomized Controlled Trial. JMIR Res Protoc 2017;6:e113. [Crossref] [PubMed]
- Ludwig SM, Waitzman KA. Changing feeding documentation to reflect infant-driven feeding practice. Newborn Infant Nurs Rev 2007;7:155-60. [Crossref]
- Waitzman KA, Ludwig SM, Nelson CLA. Contributing to content validity of the infant-driven feeding scales© through Delphi surveys. Newborn Infant Nurs Rev 2014;14:88-91. [Crossref]
- Eilers PHC, Boelens HFM. Baseline correction with asymmetric least squares smoothing. Leiden: Leiden University Medical Centre Report, 2005.
- Smith SW. FFT convolution. Digital Signal Processing 2003;
- Suhas S, Vijendra S, Burk J, et al. Spectral analysis of R wave attenuation and heart rate variability for detection of Cheyne stokes breathing. Conf Proc IEEE Eng Med Biol Soc 2005;2005:1216-9. [Crossref] [PubMed]
- Eshel G. The yule walker equations for the AR coefficients. Internet Resource 2003;2:68-73.
- Caliński T, Harabasz J. A dendrite method for cluster analysis. Commun Stat Theory Methods 1974;3:1-27. [Crossref]
- Davies DL, Bouldin DW. A cluster separation measure. IEEE Trans Pattern Anal Mach Intell 1979;1:224-7. [Crossref] [PubMed]
- Ray S, Turi RH. Determination of number of clusters in k-means clustering and application in colour image segmentation. In: Proceedings of the 4th international conference on advances in pattern recognition and digital techniques. 1999:137-43.
- Genolini C, Alacoque X, Sentenac M, et al. kml and kml3d: R packages to cluster longitudinal data. J Stat Softw 2015;65:1-34. [Crossref]
- Den Teuling NGP, Pauws SC, van den Heuvel ER. A comparison of methods for clustering longitudinal data with slowly changing trends. Commun Stat Simul Comput 2023;52:621-48. [Crossref]
- R Core Team. R: A language and environment for statistical computing. R Foundation for Statistical Computing. 2019. Available online: https://www.r-project.org/
- SAS Institute. SAS/STAT 9.4 user’s guide. (2002-2012). Available online: https://www.sas.com/
- Minitab. Minitab v19.2020.1. Available online: https://www.minitab.com/
- Martens A, Hines M, Zimmerman E. Changes in non-nutritive suck between 3 and 12 months. Early Hum Dev 2020;149:105141. [Crossref] [PubMed]
- Zimmerman E, Carpenito T, Martens A. Changes in infant non-nutritive sucking throughout a suck sample at 3-months of age. PLoS One 2020;15:e0235741. [Crossref] [PubMed]
- Morton S, Honda T, Zimmerman E, et al. Non-nutritive suck and airborne metal exposures among Puerto Rican infants. Sci Total Environ 2021;789:148008. [Crossref] [PubMed]
- Zimmerman E, Watkins DJ, Huerta-Montanez G, et al. Associations of gestational phthalate exposure and non-nutritive suck among infants from the Puerto Rico Testsite for Exploring Contamination Threats (PROTECT) birth cohort study. Environ Int 2021;152:106480. [Crossref] [PubMed]
- Maron JL. Insights into Neonatal Oral Feeding through the Salivary Transcriptome. Int J Pediatr 2012;2012:195153. [Crossref] [PubMed]
- Maron JL, Johnson KL, Dietz JA, et al. Neuropeptide Y2 receptor (NPY2R) expression in saliva predicts feeding immaturity in the premature neonate. PLoS One 2012;7:e37870. [Crossref] [PubMed]
Cite this article as: Barlow SM, Liao C, Lee J, Kim S, Maron JL, Song D, Jegatheesan P, Govindaswami B, Wilson BJ, Bhakta K, Cleary JP. Spectral features of non-nutritive suck dynamics in extremely preterm infants. Pediatr Med 2023;6:23.