Application of artificial intelligence (AI) and machine learning (ML) in pediatric epilepsy: a narrative review
Introduction
Artificial intelligence (AI) is a growing area of medical research, and the situation is the same with pediatric epilepsy (1,2). It has been actively applied to medical big data to aid various clinical practice aspects such as diagnosis, clinical treatment decision support, risk assessment, prognosis prediction, and even public health policy planning (3). Even though pediatricians are not experts in AI, it is helpful to know the current trend and recent progress made using AI in our clinical expertise. This review will present the AI application cases to pediatric epilepsy with particular emphasis on clinical significance and its practical implication rather than algorithm and methodology itself. We present the following article in accordance with the Narrative Review reporting checklist (available at https://pm.amegroups.com/article/view/10.21037/pm-21-26/rc).
Epilepsy
Epilepsy is one of the most common chronic neurological disorders in the pediatric population (4). Unlike most pediatric diseases are acute illnesses, epilepsy is a chronic condition that requires long-duration medical care and even life-long treatment. Pediatric Epileptologists should treat epilepsy in children and adolescents with care because these periods are critical to their cognitive development and educational achievements. It is well recognized that behavioral, cognitive, and psychiatric comorbidities are not uncommon in pediatric epilepsy, and they pose significant concern during their treatment (5). Epileptic seizures are recently classified as focal, generalized, and unknown (6). There are even more various epilepsy syndromes and different types of epilepsy and their etiologies are classified as structural, genetic, infectious, metabolic, immune, and unknown (7).
Seizures are highly detrimental in that they can cause fall, trauma, or even death. Seizures that do not stop spontaneously (status epilepticus) can lead to brain damage that can even cause permanent disability. Patients and their caregivers have a great fear of seizures because it is unpredictable. They are worried about the seizure attacks that occur abruptly. It is well recognized that there is a risk of sudden death in patients with epilepsy (SUDEP, sudden unexpected death of epilepsy patients) (8,9).
Epilepsy is not a single disease but a group of various entities that share the common feature of recurrent seizures. It is a very heterogeneous group of multiple diagnostic entities. The prognosis varies from one epilepsy to another in that there is self-limited epilepsy, whereas one-third or fourth of epilepsy patients experience medication refractoriness and uncontrolled daily seizure. The purpose of medical treatment of epilepsy is the prevention of seizures without significant medication adverse effects. Seizure freedom for at least 2–3 years is required for medication withdrawal, and some epilepsy patients require lifelong treatment. Monitoring medication adverse effects are crucial for long-term treatment.
AI
AI refers to any technic or program that mimics human intelligence or behavior. Machine learning is a subset of AI technology that utilizes learning from labeled or unlabeled data to build systems that have the function to learn and improve from experience automatically. Learning is the critical feature in machine learning (ML) when other AI uses specific programming to mimic human intelligence. When the algorithm is trained with the labeled dataset, it is called supervised learning. When it is trained with the data that is not labeled, it is called unsupervised learning. Deep learning is a subset of ML, which uses neural network models with many layers that resemble the human brain to generate an AI system (Figure 1).
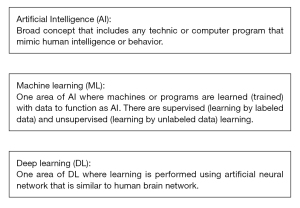
The concept of AI was coined in the 1950s when people imagined if a machine could have intelligence (10). However, it took several decades for a computer system which had sufficient computing powers to support AI technology (11). Even after AI was utilized in engineering and other science fields, the application of AI in the medical field was slow due to a lack of sufficient amount of high-quality data for training in ML (12,13). With development of digital electronic health record, digitalized biological signal data such as electroencephalography and big data such as MRI, AI in medicine showed rapid growth and achievements (14).
To name some of the AI's strengths in the medical field, one is that AI or machines are free from fatigue. Unlike humans, they are not fatigued, and as long as they are correctly programmed, they are not prone to human errors. Computer science and hardware’s advancement made a tremendous jump in computing power, which has made AI algorithms handle enormous-sized big data rapidly. As long as the algorithm is correct, AI does not have a gray zone to call for a given classification task.
AI application in epilepsy
AI has been applied to nearly all aspects of medical practice, from diagnosis to outcome prediction. It is well known that AI/ML algorithms showed equal or better performance compared to human interpreters in specific imaging diagnoses (15-18). Natural language processing and text-mining is used and has shown promising result such as gene discovery (19). It has also been applied in predicting risks of a particular disease, treatment responses, and outcomes. With the recent development of wearable devices and other health-related tracking devices, there are increasing attempts to incorporated this digitized big data to improve the management of chronic diseases (20,21).
Like other medicine fields, AI/ML was applied to various aspects of epilepsy care from epilepsy diagnosis, classification using big data such as EEG, MRI, or even medical records (Table 1). Analyzing digitized EEG and MRI images with AI to classify certain condition is one area where AI research has yielded promising results. Since epilepsy is classified as lesional and nonlesional epilepsy, identifying a causative lesion is critical regarding the treatment response, prognosis, and even candidacy of surgery in medication refractoriness. Some researchers detected and characterized focal lesions using AI (22-24). Advancing from just detecting lesions, many researchers tried to look deeper and analyze further to reveal the difference between epilepsy patients and normal controls. They used functional MRI connectivity or diffusion tensor imaging with AI to classify between epilepsy or normal control, and whether the medication response will be good or bad (25-27). Medical records such as progress notes and EEG reports were analyzed with AI (28-30).
Table 1
Epilepsy care | Data used | Examples |
---|---|---|
Diagnosis | EEG | Automated EEG analysis |
Interictal epileptiform discharge detection | ||
MRI | Classifying MRI with epilepsy vs. without epilepsy | |
Epilepsy causative lesions detection | ||
EHR | Automatically analyze progress note or EEG report | |
Treatment | EHR | Algorithm suggesting antiseizure medication |
MRI, EEG | Epileptogenic zone detection | |
Seizure detection and prediction | EEG | Seizure detection and prediction using scalp EEG |
Seizure detection and prediction using intracranial EEG | ||
Other sensors | Seizure detection and prediction using EMG, motion detector and other sensors | |
Outcome prediction | EHR | Overall epilepsy outcome prediction |
MRI | Seizure and non-seizure epilepsy outcome prediction |
EEG, electroencephalogram; MRI, magnetic resonance imaging; EHR, electronic health record; EMG, electromyogram.
Since seizure control is the ultimate goal in epilepsy treatment, seizure detection is a crucial issue. Moreover, seizure prediction and forecasting are other promising fields that can directly help patients improve their quality of life. There are a large number of studies regarding seizure detection using EEG (31) and recent studies utilized videos and motion sensors to detect and classify seizures (32,33). These efforts were even applied to challenging fields such as neonatal seizure (34).
The application of AI to predict treatment response for treatment like antiseizure medication and epilepsy surgery is also a critical issue. Some studies tried to analyze various clinical features and phenotypes to predict treatment response (35). Others have tried to predict medication refractoriness even at the time of initial treatment (36). As well as treatment response prediction, many studies attempted to predict outcomes other than seizure control which are relevant to epilepsy patients. Such outcomes are cognitive functioning such as language dysfunction, whether patients are provided with adequate antiseizure medication, and emergency department visits during their treatment (37-39). Another area of interesting and clinically useful research is prediction surgical outcome. Epilepsy surgery is one of the last resorts for epilepsy patients with medication refractoriness, and it is a procedure with possible surgical complications and functional deficit. AI research has helped plan the surgery to accurately localize the resection zone and predict surgical outcome after surgery (40-43).
Interpretation of electroencephalogram (EEG)
Interpretation of EEG is very complicated. Various artifacts, features of unknown significance, benign variants, and delicate fluctuation are some of the issues making EEG interpretation such a technically challenging task. While the quantitative analysis was one methodology that enabled technical advancement in image processing (various medical images such as magnetic resonance image (MRI), simple radiographs, and endoscopy images), EEG was not the easily accessed clinical data for AI. Japanese researchers did pioneering work for more than thirty years to build a computer-assisted system to interpret adult EEG automatically (44-46). They made a system to analyze EEG to meet electroencephalographer's visual inspection and to detect artifacts, spikes automatically, and vigilance and attention level of individual subject for the final purpose to supplement visual inspection. We are also building an AI implement routine EEG analysis software as a South Korean Government-funded project. This software's primary purpose is to automatically detect analysis epochs, perform quantitative analysis to aid visual interpretation, and finally build a structured database of quantitative features of EEG (47).
Awake sleep cycle staging is a specific field where AI/ML has showed promising results. When we interpret EEG and polysomnography, sleep-wake cycle staging is an initial and essential step. There have been many attempts to classify wake-sleep staging with EEG to yield good accuracy (48-50). We developed a multidomain hybrid neural network and classified pediatric EEG solely using EEG alone to yield similar accuracy than previous studies with adult EEG. As clinicians, we took a further step to test this algorithm for various parameters. We aimed to determine whether there are differences in performance depending on epoch length (10, 20, and 30 seconds) and the number of channels (whole 19 channels vs. the reduced number that is the same as in PSG). The best result came with 30 seconds with the entire 19channel, but the difference in reducing the number and shortening the epoch length was less than 3% (51). It is an example where clinicians working together with AI researchers can test hypotheses based on clinical experience and yield study outcomes with practical clinical implications.
Spike detection is another field where AI is used in epilepsy diagnosis. There are many different kinds of interictal epileptiform discharges, such as spike, spike and wave, sharp wave, polyspike and waves. Their morphology differs regarding amplitude, orientation, location, and occurrence. Researchers used to count these spikes from paper EEG, and it was very tedious and labor-intensive work. Even though this was done manually in the research setting, it is seldom possible in clinical settings. Some studies tried to detect and classify these automatically discharges to help improve EEG interpretation and analysis from the scalp and intracranial EEGs (52-56).
Seizure detection and forecasting using EEG
Epilepsy patients undergo long-term EEG monitoring for multiple purposes such as pattern evaluation and presurgical workup. An increasing number of continuous monitoring in intensive care units are performed for status epilepticus management and other neurocritical care. Seizure detection is an essential step in interpreting these study results and timely management to improve clinical outcomes. Moreover, data from devices such as response neurostimulator (RNS) and other wearable devices are promising materials for training in AI/ML to detect and predict seizures. Successful forecasting eventually can lower anticipation for patients or their caregivers and even improve seizure control.
Since 1970s effort to detect seizure took place and it has developed to the stage that use AI algorithms to detect spontaneous seizure attacks (57-59). Seizure detection in AI means classifying the specific epoch of EEG as a seizure or non-seizure epoch. EEG epochs are labeled as seizure or non-seizure, and these EEGs are used for training and validation. Whereas in seizure prediction or forecasting, epochs are classified as interictal (between seizure attacks) and preictal (before the seizure for a certain amount of time). AI algorithms are trained to classify and detect the preictal epoch. In addition to accuracy, the false positive rate is an essential measure of performance. In case of high false positive alarms, the patient will have to anticipate seizure unnecessarily, and lowering this false alarm rate is relevant. Many studies in seizure prediction and the sensitivity and specificity have increased remarkably for few decades (60-63).
We developed deep convolutional neural network-based interictal/preictal EEG prediction and applied to 9 pediatric patients with surgically proven focal cortical dysplasia type II. The best accuracy was 5 minutes as preictal period, all intracranial channels for analysis, and 512 Hz sampling rate for EEG acquisition. When we change the preictal period from 2 hours before seizure to 1 minute, accuracy increased to 5 minutes and showed a small decline in 1 minute. These findings tell us that the best functioning was when we set the preictal period to 5 minutes before the seizure started. When we changed the analyzing electrode from whole intracranial to most relevant four electrodes, accuracy declined, but the amount was 2%. Increasing the sampling rate from 128 to 512 Hz, the increase of accuracy was trivial. We found that we could reduce the number of electrodes and sampling rate with a slight decline in performance. The decreasing number of electrodes helps reduce surgical risk, and sampling rate reduction is related to computational efficiency (64).
Limitation and suggestions
AI is a growing field of science that evidently is changing the landscape of medicine and pediatric epilepsy. However, there are limitations to this cutting-edge technology. Especially in ML, it is totally dependent on the quality of training data. It is even true that inadequate quality labeling or wrongly labeled training datasets will end up with poorly functioning or wrongfully directing algorithms trained by supervised learning. Labeling is very labor-intensive, and it requires effort to have a high-quality training dataset.
Above mentioned limitations are the reasons why pediatricians need to be aware of AI technology. To improve the AI/ML algorithm’s quality, practicing clinicians should correctly label the data with their expertise. The algorithm must be designed carefully with the insight of clinicians and their practical needs. AI algorithm and advancement of computer technology is not threatening human doctors. They need human doctors' help and understanding to improve the algorithm and further functioning. Eventually, AI technology advancement and the wide application of AI in medicine will improve medical care quality.
Acknowledgments
Funding: None.
Footnote
Reporting Checklist: The authors have completed the Narrative Review reporting checklist. Available at https://pm.amegroups.com/article/view/10.21037/pm-21-26/rc
Conflicts of Interest: Both authors have completed the ICMJE uniform disclosure form (available at https://pm.amegroups.com/article/view/10.21037/pm-21-26/coif). The authors have no conflicts of interest to declare.
Ethical Statement: The authors are accountable for all aspects of the work in ensuring that questions related to the accuracy or integrity of any part of the work are appropriately investigated and resolved.
Open Access Statement: This is an Open Access article distributed in accordance with the Creative Commons Attribution-NonCommercial-NoDerivs 4.0 International License (CC BY-NC-ND 4.0), which permits the non-commercial replication and distribution of the article with the strict proviso that no changes or edits are made and the original work is properly cited (including links to both the formal publication through the relevant DOI and the license). See: https://creativecommons.org/licenses/by-nc-nd/4.0/.
References
- He J, Baxter SL, Xu J, et al. The practical implementation of artificial intelligence technologies in medicine. Nat Med 2019;25:30-6. [Crossref] [PubMed]
- Fogel AL, Kvedar JC. Artificial intelligence powers digital medicine. NPJ Digit Med 2018;1:5. [Crossref] [PubMed]
- Schwalbe N, Wahl B. Artificial intelligence and the future of global health. Lancet 2020;395:1579-86. [Crossref] [PubMed]
- Russ SA, Larson K, Halfon N. A national profile of childhood epilepsy and seizure disorder. Pediatrics 2012;129:256-64. [Crossref] [PubMed]
- Nickels KC, Zaccariello MJ, Hamiwka LD, et al. Cognitive and neurodevelopmental comorbidities in paediatric epilepsy. Nat Rev Neurol 2016;12:465-76. [Crossref] [PubMed]
- Fisher RS, Cross JH, French JA, et al. Operational classification of seizure types by the International League Against Epilepsy: Position Paper of the ILAE Commission for Classification and Terminology. Epilepsia 2017;58:522-30. [Crossref] [PubMed]
- Scheffer IE, Berkovic S, Capovilla G, et al. ILAE classification of the epilepsies: Position paper of the ILAE Commission for Classification and Terminology. Epilepsia 2017;58:512-21. [Crossref] [PubMed]
- Sveinsson O, Andersson T, Carlsson S, et al. The incidence of SUDEP: A nationwide population-based cohort study. Neurology 2017;89:170-7. [Crossref] [PubMed]
- Annegers JF, Coan SP. SUDEP: overview of definitions and review of incidence data. Seizure 1999;8:347-52. [Crossref] [PubMed]
- Ramesh AN, Kambhampati C, Monson JR, et al. Artificial intelligence in medicine. Ann R Coll Surg Engl 2004;86:334-8. [Crossref] [PubMed]
- Kaul V, Enslin S, Gross SA. History of artificial intelligence in medicine. Gastrointest Endosc 2020;92:807-12. [Crossref] [PubMed]
- Raju B, Jumah F, Ashraf O, et al. Big data, machine learning, and artificial intelligence: a field guide for neurosurgeons. J Neurosurg 2020; Epub ahead of print. [Crossref] [PubMed]
- Auger SD, Jacobs BM, Dobson R, et al. Big data, machine learning and artificial intelligence: a neurologist's guide. Pract Neurol 2020; Epub ahead of print. [Crossref] [PubMed]
- Abbasi B, Goldenholz DM. Machine learning applications in epilepsy. Epilepsia 2019;60:2037-47. [Crossref] [PubMed]
- Mnih V, Kavukcuoglu K, Silver D, et al. Human-level control through deep reinforcement learning. Nature 2015;518:529-33. [Crossref] [PubMed]
- Russakovsky O, Deng J, Su H, et al. ImageNet Large Scale Visual Recognition Challenge. Int J Comput Vision 2015;115:211-52. [Crossref]
- Esteva A, Kuprel B, Novoa RA, et al. Dermatologist-level classification of skin cancer with deep neural networks. Nature 2017;542:115-8. [Crossref] [PubMed]
- Gulshan V, Peng L, Coram M, et al. Development and Validation of a Deep Learning Algorithm for Detection of Diabetic Retinopathy in Retinal Fundus Photographs. JAMA 2016;316:2402-10. [Crossref] [PubMed]
- Lüscher Dias T, Schuch V, Beltrão-Braga PCB, et al. Drug repositioning for psychiatric and neurological disorders through a network medicine approach. Transl Psychiatry 2020;10:141. [Crossref] [PubMed]
- Wasserlauf J, You C, Patel R, et al. Smartwatch Performance for the Detection and Quantification of Atrial Fibrillation. Circ Arrhythm Electrophysiol 2019;12:e006834. [Crossref] [PubMed]
- Hafezi M, Montazeri N, Zhu K, et al. Sleep Apnea Severity Estimation from Respiratory Related Movements Using Deep Learning. Annu Int Conf IEEE Eng Med Biol Soc 2019;2019:1601-4. [Crossref] [PubMed]
- El Azami M, Hammers A, Jung J, et al. Detection of Lesions Underlying Intractable Epilepsy on T1-Weighted MRI as an Outlier Detection Problem. PLoS One 2016;11:e0161498. [Crossref] [PubMed]
- Hong SJ, Bernhardt BC, Schrader DS, et al. Whole-brain MRI phenotyping in dysplasia-related frontal lobe epilepsy. Neurology 2016;86:643-50. [Crossref] [PubMed]
- Jin B, Krishnan B, Adler S, et al. Automated detection of focal cortical dysplasia type II with surface-based magnetic resonance imaging postprocessing and machine learning. Epilepsia 2018;59:982-92. [Crossref] [PubMed]
- Zhang J, Cheng W, Wang Z, et al. Pattern classification of large-scale functional brain networks: identification of informative neuroimaging markers for epilepsy. PLoS One 2012;7:e36733. [Crossref] [PubMed]
- Amarreh I, Meyerand ME, Stafstrom C, et al. Individual classification of children with epilepsy using support vector machine with multiple indices of diffusion tensor imaging. Neuroimage Clin 2014;4:757-64. [Crossref] [PubMed]
- Soriano MC, Niso G, Clements J, et al. Automated Detection of Epileptic Biomarkers in Resting-State Interictal MEG Data. Front Neuroinform 2017;11:43. [Crossref] [PubMed]
- Connolly B, Matykiewicz P, Bretonnel Cohen K, et al. Assessing the similarity of surface linguistic features related to epilepsy across pediatric hospitals. J Am Med Inform Assoc 2014;21:866-70. [Crossref] [PubMed]
- Connolly B, Matykiewicz P. Assessing the similarity of surface linguistic features related to epilepsy across pediatric hospitals. J Am Med Inform Assoc 2014;21:866-70. [Crossref] [PubMed]
- Goodwin TR, Harabagiu SM. Multi-modal Patient Cohort Identification from EEG Report and Signal Data. AMIA Annu Symp Proc 2016;2016:1794-803. [PubMed]
- Acharya UR, Hagiwara Y, Adeli H. Automated seizure prediction. Epilepsy Behav 2018;88:251-61. [Crossref] [PubMed]
- Karayiannis NB, Xiong Y, Tao G, et al. Automated detection of videotaped neonatal seizures of epileptic origin. Epilepsia 2006;47:966-80. [Crossref] [PubMed]
- Onorati F, Regalia G, Caborni C, et al. Multicenter clinical assessment of improved wearable multimodal convulsive seizure detectors. Epilepsia 2017;58:1870-9. [Crossref] [PubMed]
- Karayiannis NB, Tao G, Xiong Y, et al. Computerized motion analysis of videotaped neonatal seizures of epileptic origin. Epilepsia 2005;46:901-17. [Crossref] [PubMed]
- Aslan K, Bozdemir H, Sahin C, et al. Can neural network able to estimate the prognosis of epilepsy patients according to risk factors? J Med Syst 2010;34:541-50. [Crossref] [PubMed]
- An S, Malhotra K, Dilley C, et al. Predicting drug-resistant epilepsy - A machine learning approach based on administrative claims data. Epilepsy Behav 2018;89:118-25. [Crossref] [PubMed]
- Piña-Garza JE, Montouris GD, Vekeman F, et al. Assessment of treatment patterns and healthcare costs associated with probable Lennox-Gastaut syndrome. Epilepsy Behav 2017;73:46-50. [Crossref] [PubMed]
- Paldino MJ, Zhang W, Chu ZD, et al. Metrics of brain network architecture capture the impact of disease in children with epilepsy. Neuroimage Clin 2017;13:201-8. [Crossref] [PubMed]
- Grinspan ZM, Patel AD, Hafeez B, et al. Predicting frequent emergency department use among children with epilepsy: A retrospective cohort study using electronic health data from 2 centers. Epilepsia 2018;59:155-69. [Crossref] [PubMed]
- Grigsby J, Kramer RE, Schneiders JL, et al. Predicting outcome of anterior temporal lobectomy using simulated neural networks. Epilepsia 1998;39:61-6. [Crossref] [PubMed]
- Arle JE, Perrine K, Devinsky O, et al. Neural network analysis of preoperative variables and outcome in epilepsy surgery. J Neurosurg 1999;90:998-1004. [Crossref] [PubMed]
- Armañanzas R, Alonso-Nanclares L, Defelipe-Oroquieta J, et al. Machine learning approach for the outcome prediction of temporal lobe epilepsy surgery. PLoS One 2013;8:e62819. [Crossref] [PubMed]
- Roland JL, Griffin N, Hacker CD, et al. Resting-state functional magnetic resonance imaging for surgical planning in pediatric patients: a preliminary experience. J Neurosurg Pediatr 2017;20:583-90. [Crossref] [PubMed]
- Nakamura M, Shibasaki H, Imajoh K, et al. Automatic EEG interpretation: a new computer-assisted system for the automatic integrative interpretation of awake background EEG. Electroencephalogr Clin Neurophysiol 1992;82:423-31. [Crossref] [PubMed]
- Nakamura M, Sugi T, Ikeda A, et al. Clinical application of automatic integrative interpretation of awake background EEG: quantitative interpretation, report making, and detection of artifacts and reduced vigilance level. Electroencephalogr Clin Neurophysiol 1996;98:103-12. [Crossref] [PubMed]
- Shibasaki H, Nakamura M, Sugi T, et al. Automatic interpretation and writing report of the adult waking electroencephalogram. Clin Neurophysiol 2014;125:1081-94. [Crossref] [PubMed]
- Shibasaki H, Nakamura M. Automatic integrated EEG interpretation and reporting. In: Schomer D, Lopes da Silva F, editors. Niedermeyer's Electroencephalography. New York: Oxford University Press; 2018. p. 714-20.
- Diykh M, Li Y. Complex networks approach for EEG signal sleep stages classification. Expert Syst Appl 2016;63:241-8. [Crossref]
- Hassan AR, Bhuiyan MIH. Automated identification of sleep states from EEG signals by means of ensemble empirical mode decomposition and random under sampling boosting. Comput Methods Programs Biomed 2017;140:201-10. [Crossref] [PubMed]
- Chriskos P, Frantzidis CA, Gkivogkli PT, et al. Achieving Accurate Automatic Sleep Staging on Manually Pre-processed EEG Data Through Synchronization Feature Extraction and Graph Metrics. Front Hum Neurosci 2018;12:110. [Crossref] [PubMed]
- Jeon Y, Kim S, Choi HS, et al. Pediatric Sleep Stage Classification Using Multi-Domain Hybrid Neural Networks. IEEE Access 2019;7:96495-505.
- Antoniades A, Spyrou L, Martin-Lopez D, et al. Detection of Interictal Discharges With Convolutional Neural Networks Using Discrete Ordered Multichannel Intracranial EEG. IEEE Trans Neural Syst Rehabil Eng 2017;25:2285-94. [Crossref] [PubMed]
- Tjepkema-Cloostermans MC, de Carvalho RCV, van Putten MJAM. Deep learning for detection of focal epileptiform discharges from scalp EEG recordings. Clin Neurophysiol 2018;129:2191-6. [Crossref] [PubMed]
- Fürbass F, Kural MA, Gritsch G, et al. An artificial intelligence-based EEG algorithm for detection of epileptiform EEG discharges: Validation against the diagnostic gold standard. Clin Neurophysiol 2020;131:1174-9. [Crossref] [PubMed]
- Jing J, Sun H, Kim JA, et al. Development of Expert-Level Automated Detection of Epileptiform Discharges During Electroencephalogram Interpretation. JAMA Neurol 2020;77:103-8. [Crossref] [PubMed]
- Abou Jaoude M, Jing J, Sun H, et al. Detection of mesial temporal lobe epileptiform discharges on intracranial electrodes using deep learning. Clin Neurophysiol 2020;131:133-41. [Crossref] [PubMed]
- Shoeb A, Carlson D, Panken E, et al. A micropower support vector machine based seizure detection architecture for embedded medical devices. Annu Int Conf IEEE Eng Med Biol Soc 2009;2009:4202-5. [Crossref] [PubMed]
- Kharbouch A, Shoeb A, Guttag J, et al. An algorithm for seizure onset detection using intracranial EEG. Epilepsy Behav 2011;22:S29-35. [Crossref] [PubMed]
- Ansari AH, Cherian PJ, Dereymaeker A, et al. Improved multi-stage neonatal seizure detection using a heuristic classifier and a data-driven post-processor. Clin Neurophysiol 2016;127:3014-24. [Crossref] [PubMed]
- Wang SY, Chaovalitwongse WA, Wong S. Online Seizure Prediction Using an Adaptive Learning Approach. IEEE T Knowl Data En 2013;25:2854-66. [Crossref]
- Moghim N, Corne DW. Predicting epileptic seizures in advance. PLoS One 2014;9:e99334. [Crossref] [PubMed]
- Cho D, Min B, Kim J, et al. EEG-Based Prediction of Epileptic Seizures Using Phase Synchronization Elicited from Noise-Assisted Multivariate Empirical Mode Decomposition. IEEE Trans Neural Syst Rehabil Eng 2017;25:1309-18. [Crossref] [PubMed]
- Jacobs D, Hilton T, del Campo M, et al. Classification of Pre-Clinical Seizure States Using Scalp EEG Cross-Frequency Coupling Features. IEEE T Bio-Med Eng 2018;65:2440-9.
- Chung YG, Jeon Y, Choi SA, et al. Deep Convolutional Neural Network Based Interictal-Preictal Electroencephalography Prediction: Application to Focal Cortical Dysplasia Type-II. Front Neurol 2020;11:594679. [Crossref] [PubMed]
Cite this article as: Kim H, Hwang H. Application of artificial intelligence (AI) and machine learning (ML) in pediatric epilepsy: a narrative review. Pediatr Med 2022;5:24.